引用本文: | 戴渝心,马毅,姜宗辰,杜凯,王海起.基于多核SVM决策融合模型的海面原油多光谱遥感检测研究[J].海洋科学,2022,46(7):11-23. |
| |
|
|
|
本文已被:浏览 636次 下载 1220次 |
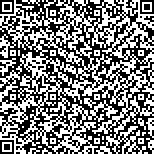 码上扫一扫! |
|
基于多核SVM决策融合模型的海面原油多光谱遥感检测研究 |
戴渝心1,2, 马毅2,3, 姜宗辰4, 杜凯5, 王海起1
|
1.中国石油大学(华东)海洋与空间信息学院, 山东 青岛 266580;2.自然资源部第一海洋研究所, 山东 青岛 266061;3.自然资源部海洋遥测技术创新中心, 山东 青岛 266061;4.哈尔滨工业大学, 黑龙江 哈尔滨 150001;5.山东科技大学, 山东 青岛 266590
|
|
摘要: |
海面溢油事故发生后需要进行精准的溢油检测,从而为溢油事故现场应急响应与海面污染的快速有效处理提供支持。利用哨兵二号(Sentinel-2)多光谱遥感影像,基于最佳指数因子(OIF)构建光谱特征指数提取海面溢油光谱特征,基于灰度共生矩阵(GLCM)提取海面溢油纹理特征,利用巴氏距离法进行特征选择,构建海面溢油空谱特征数据集。提出了多核决策融合支持向量机(SVM)海面原油检测模型,对黄海4.27“交响乐号”油轮溢油事故开展检测研究。实验结果表明,在复杂水色背景下,经过筛选的最佳空谱特征数据集检测结果的总体精度可以达到89.25%,Kappa系数为0.854,F1分数可达0.889;多核SVM决策融合后的总体精度可以达到90.26%,Kappa系数为0.866,F1可达0.898,总体精度较单核提高了1.0%~8.2%,Kappa系数提高了0.013~0.122,F1分数提高了0.009~0.097。实验结果表明,海面溢油检测模型具有较高的溢油检测精度和模型鲁棒性,适用于海面溢油检测研究。 |
关键词: 遥感 溢油检测 特征提取 支持向量机 决策融合 |
DOI:10.11759/hykx20210824001 |
分类号:P76 |
基金项目:国家自然科学基金重大项目(61890964);山东省联合基金项目(U1906217) |
|
Multi-spectral remote sensing detection of marine oil spill based on multi-kernel SVM decision fusion model |
DAI Yu-xin1,2, MA Yi2,3, JIANG Zong-chen4, DU Kai5, WANG Hai-qi1
|
1.College of Oceanography and Space Informatics, China University of Petroleum (East China), Qingdao 266580, China;2.First Institute of Oceanology, Ministry of Natural Resources, Qingdao 266061, China;3.Technology Innovation Center for Ocean Telemetry, Ministry of Natural Resources, Qingdao 266061, China;4.Harbin Institute of Technology, Harbin 150001, China;5.Shandong University of Science and Technology, Qingdao 266590, China
|
Abstract: |
After an oil spill accident, accurate oil spill detection is needed to support the on-site emergency response to the accident and the rapid and effective treatment of sea surface pollution. In this paper, a sentinel-2 multispectral remote sensing image is used to construct spectral feature indices based on the OIF index to extract the spectral features of oil spills on the sea surface. The texture features of oil spills are extracted based on the gray level co-occurrence matrix (GLCM). The feature selection method is used to construct an optimal texture and spectral feature dataset of oil spills on the sea surface. In this paper, a multi-core decision fusion support vector machine (SVM) model is proposed to detect the oil spill accident of the "A Symphony" tanker in the Yellow Sea. The experimental results show that under the complex water-color background, the detection results of the optimal texture and spectral feature dataset reach 89.25%, the Kappa coefficient is 0.854, and the F1-score is 0.889. After multi-kernel SVM decision fusion, the OA, Kappa coefficient, and F1-score reach 90.26%, 0.866, and 0.898, respectively. The OA, Kappa coefficient, and F1-score increase by 1.0%-8.2%, 0.013-0.122, and 0.009-0.097, respectively, compared with those of the single-kernel SVM. The experimental results show that the proposed oil spill detection model has high accuracy and robustness, and the proposed method is suitable for oil spill detection. |
Key words: remote sensing oil spill detection feature extraction support vector machine (SVM) decision fusion |
|
|
|
|
|
|